Propensity models are a group of statistical models that provide immense value to your organization.
Read MoreCustomer Propensity Modeling
Propensity is the natural tendency to behave in a particular way. Using that definition it should be easy to see customer propensity modeling is the use of predictive models to try and predict customer behavior. Understanding these behaviors can allow your business to positively impact your relationship with your customers.
With a general understanding of what propensity modeling is, the next step is to define some behaviors that can be modeled. Below are some common examples of types of propensity models used in marketing analytics.
LTV – LTV propensity models look to predict the value of a customer. This can be done at any time in the customer lifecycle. A customer’s LTV can be a useful tool in segmenting your customers. More effort then can be used in starting a relationship with more valuable customers.
Likelihood to Buy – This propensity model tries to predict when a customer is likely to buy. This model may look at data points like website visits, marketing touchpoints, form fills and more to identify people that are in the buying mood. Knowing a customer is in a buying mood can allow you to communicate in relevant ways to close the deal.
Churn – A customer who has churned is one that no longer has a relationship with your company. Knowing when a customer may churn can allow you to create retention programs to try and save the relationship.
Response/Engagement – Another useful propensity model is response modeling. This model predicts the likelihood of a person to respond to a marketing piece such as a mailer, email, or outbound call. This type of model is another effective way to focus limited resources on the people most likely to create a result.
The propensity models listed above are only a few examples of customer behaviors that can be modeled. Depending on the availability of data the possibilities of modeling customer behaviors can be large. Creating the model is only one step in an effective strategy. The next step is to implement the model and a program to provide action. The next section will describe one way to use propensity modeling to increase revenue for your data driven organization.
One of my favorite ways to use propensity modeling is to create a customer quadrant segmentation structure by using LTV and Churn propensity models together. The idea here is to create a quadrant to segment customers into four categories which are HIgh Value Intervention, Low Work High Value, Low Value and High Work Customers. A picture representing the quadrant can be found below.
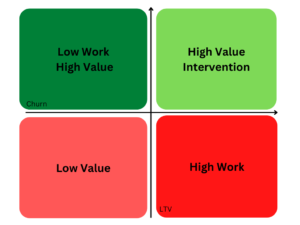
This quadrant can inform a retention strategy with treatments outlined below.
- Low Work High Value – These customers have a low propensity to churn and are high value. You should leave this group alone and let them do their thing.
- High Value Intervention – These customers need to be examined to recognize save opportunities. Unlike the low work group you will need to work to keep their business, but the high LTV should make it worth it.
- Low Value – This segment should also be left alone. Their low value is not worth focusing your limited resources on.
- High Work – This segment of customers is the most dangerous to creating profitable customer relationships. These customers do not bring value, but they will burn through resources trying to keep their business.
Of the four segments the High Value Intervention segment is the most important to focus your energy on. This type of segmentation is a good example of using the Pareto Principle to optimize your customer lifecycle management. Other propensity models can be used in similar fashions to increase profitable revenue within your business.
Related Articles We Think You Would Enjoy Next
Propensity Modeling: How to Identify and Target Likely Buyers
Understanding who is likely to purchase allows you to optimize your efforts. How do you do this?
Read MoreReporting Unleashed: Elevate Your Small Business with SMART Data Reporting
Reporting is often the most overlooked piece of data analytics. Reporting is the key cog in the data analytics.
Read Moreare you looking to utilize propensity models in your business, but are unsure how. Reach out to Capitol Data Analytics to get a free consultation